Predictive Analysis
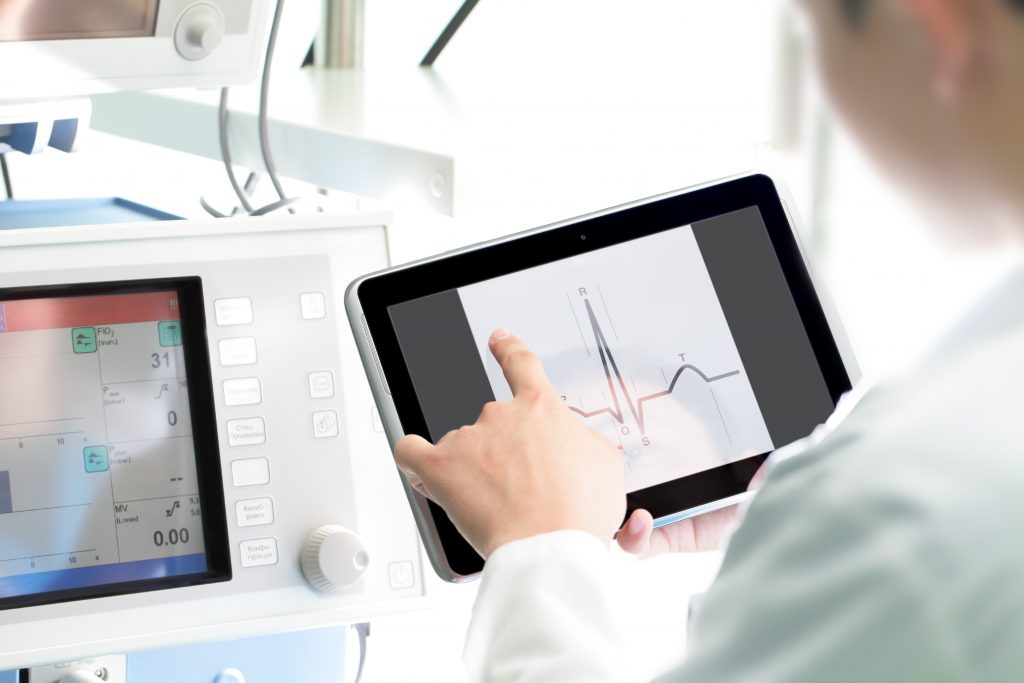
Artificial Intelligence and Predictive Analysis
AI and predictive analytics use data mining and modeling, statistics, and machine learning, to make predictions about otherwise unknowable future events. The goal is to move from what has happened to providing insight into what will happen. Healthcare organizations use AI and predictive analytics for a variety of reasons. They include: detecting fraud, improving patient outcomes, maintaining regulatory compliance, optimizing marketing efforts, improving operations, and reducing risk.
The Advis Approach to AI and Predictive Analytics:
- Define Project Scope
- Here Advis defines project outcomes, deliverables, business objections, and data sets to be used in problem analysis.
- Data Compilation
- Advis works with interdisciplinary teams to obtain and transfer any necessary data safely and securely.
- Data Analysis
- This process involves Advis inspecting, cleaning, and transforming the modeling data.
- Statistics
- Advis employs statistical analysis to validate assumptions and hypothesis.
- Modeling
- Advis builds a predictive algorithm within the best suited model for the problem set to provide insight into future events.
- Implementation
AI and Predictive Analytics may be the right solution for you if:
- You can define the business result you want to achieve;
- Determining what you want to know about the future based on the past and what decisions will be driven by the derivation of new insights is the first step to using predictive analytics.
- You have readily available historical data;
- Considered one of the most difficult steps of the predictive analytics process, data collection requires teamwork between business units and IT professionals.
Examples of Predictive Analytics in action:
- Timely Admission, Discharge, or Transfer
- Bed Utilization and Predictive Need
- One Day Stays by DRG
- Resource Allocation
- Uncompensated Care Loss
- Patterns for Discharge by Day of the Week
- Analysis of Length of Stay and Payer Class
Predictive Analytics
Predictive analytics uses data mining and modeling, statistics, machine learning, and artificial intelligence to make predictions about otherwise unknowable future events. The goal is to move from what has happened to providing insight into what will happen. Healthcare organizations use predictive analytics for a variety of reasons. They include: detecting fraud, improving patient outcomes, maintaining regulatory compliance, optimizing marketing efforts, improving operations, and reducing risk.
The Advis Approach to Predictive Analytics:
- Define Project Scope
- Here Advis defines project outcomes, deliverables, business objections, and data sets to be used in problem analysis.
- Data Compilation
- Advis works with interdisciplinary teams to obtain and transfer any necessary data safely and securely.
- Data Analysis
- This process involves Advis inspecting, cleaning, and transforming the modeling data.
- Statistics
- Advis employs statistical analysis to validate assumptions and hypothesis.
- Modeling
- Advis builds a predictive algorithm within the best suited model for the problem set to provide insight into future events.
- Implementation
Predictive Analytics may be the right solution for you if:
- You can define the business result you want to achieve;
- Determining what you want to know about the future based on the past and what decisions will be driven by the derivation of new insights is the first step to using predictive analytics.
- You have readily available historical data;
- Considered one of the most difficult steps of the predictive analytics process, data collection requires teamwork between business units and IT professionals.
Examples of Predictive Analytics in action:
- Timely Admission, Discharge, or Transfer
- Bed Utilization and Predictive Need
- One Day Stays by DRG
- Resource Allocation
- Uncompensated Care Loss
- Patterns for Discharge by Day of the Week
- Analysis of Length of Stay and Payer Class